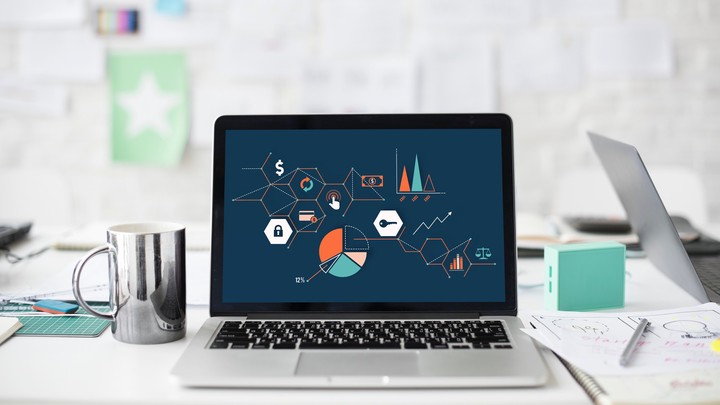
It is increasingly common for data to be collected adaptively, where experimental costs are reduced progressively by assigning promising treatments more frequently. By skewing the allocation of the arms towards the more efficient or informative ones, they have the potential to enhance participants’ welfare, while resulting in a more flexible, efficient, and ethical alternative compared to traditional studies. However, such allocation strategies complicate the problem of statistical inference. It is now recognized that traditional inference methods are typically not valid when used in adaptively-collected data (such as those driven by multi-armed bandit problems), leading to considerable biases in classical estimators and other relevant issues in hypothesis testing problems.